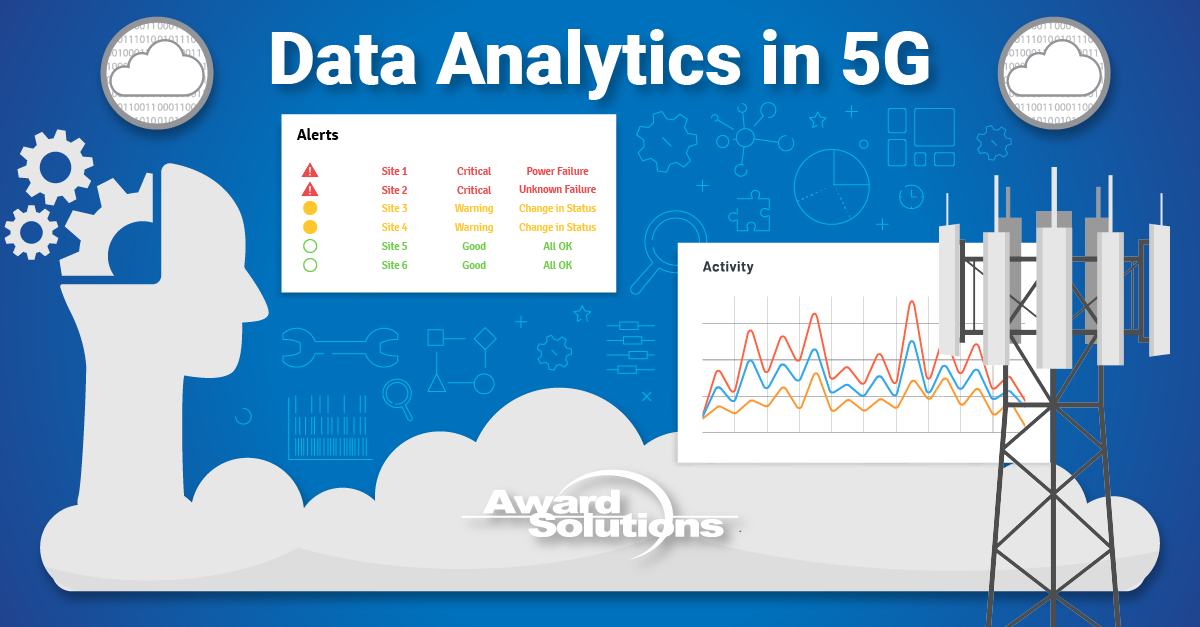
Imagine running a manufacturing facility and not using 98% of the means of production. Not a happy situation, I'm sure you'll agree. Today, less than 2% of the data generated by 4G and 5G networks is stored (not counting data consumed by the subscribers, obviously), and less than 10% of that is analyzed and used in some way. In the digital economy of our age, call it Industry 4.0 if you will, data and the insights it provides are the equivalents of the 'means of production' in addition to the machinery and the workforce of the former industrial era. The telco sector needs to change and effectively use these vast untapped resources, making them the heart of their innovations. Here, we discuss the challenges with past data-analytics efforts in 4G and how 5G networks can finally take on those challenges to take data analytics to a real advantage.
Data generation in Wireless Networks
5G is not the first telco infrastructure to attempt harnessing network-generated data for optimizing operations. Mobile Network Operators (MNOs), have been collecting and using data from the earliest days of cellular. Cellular networks generate data in different ways:
a) Data generated by the network nodes or components – the most common examples are counters and related KPIs (Key Performance Indicators), alarms, and other performance statistics in the radio and the core networks. Every CDR (Call data record) and signaling transaction can be a contributing source of data.
b) Data generated by the User Equipment (Device) – Common examples are position and mobility, radio condition metrics, and the user experience in general. A modern smartphone is essentially a never-ending fountain of ancillary data beyond the gigabytes of the data consumed by the subscriber
c) Data generated by applications and services – this data can overlap with the previous two modes, but it can also stand alone and provide additional insights. Ookla speed testing is one prominent example, collecting connection speed tests as a function of the device location and technology and later shared (or purchased) for data analytics.
Lessons from Past
In the domain of 4G, where virtualization and cloud architecture was introduced late in the game, these data streams were not harnessed effectively. An example is LTE's 3GPP-defined SON (self-optimizing and self-healing network) feature. Unfortunately, the benefits of SON have been mixed, and most agree that although it has excelled in some areas (like automatic cell neighbor relations), it has fallen short of its full potential. The reasons for this can be seen in the lack of a coherent and unified approach for collecting data and the largely monolithic architecture of 4G networks which forced the concepts of distributed and centralized SON. This fractured approach to SON in legacy 4G systems could even turn them into competing control entities, as one SON system would try to tweak changes that another SON system had forced into place. It is like your right-hand wants to turn the steering wheel in the opposite direction of what your left hand is trying to do while driving a car!
The radical design of the 5G network as a completely virtual and cloudified service-based architecture is now paving the way for overcoming past hurdles with data analytics and actionable insights. As a result, the boundaries between the telco and cloud companies are blurrier than ever, allowing the cloud mentality and a data-centric approach. Let us look closer at the 5G network and its data analytics function.
5G Core and the NWDAF
In many ways, the 5G core is an even greater break from the legacy 4G than the radio network is with its use of mmWaves. Gone are the monoliths of MME, SGW, PGW, PCRF, and the rest with their plethora of esoteric protocols like Diameter and GTPv2-C. Instead, the 5G Core is designed for a cloud environment where essentially all network functions (NFs) are virtual, disaggregated, and conform to the Service Based Paradigm, where the main protocol is HTTP/2, and the instantiated network function runs on the same hardware platform used in data centers and clouds.
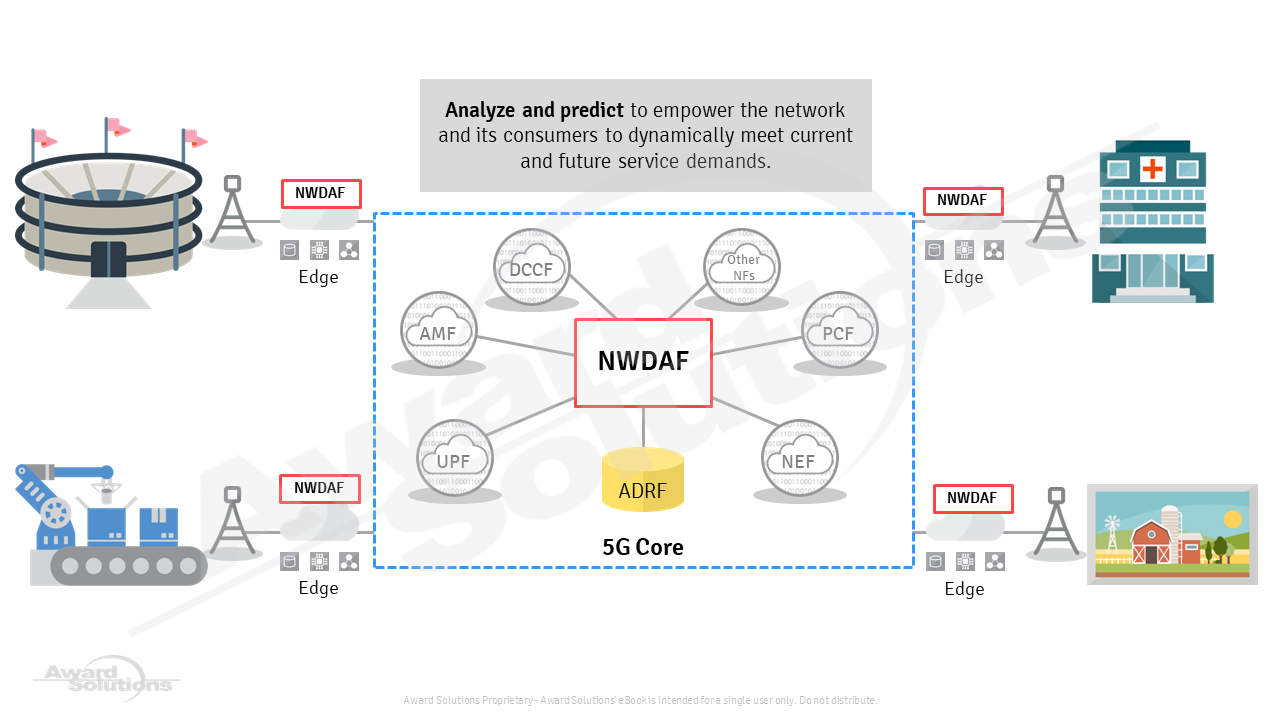
With this new networking paradigm, the 3GPP specifications have introduced the NWDAF (Network Data Analytics Function). The NWDAF function in the 5G core collects data from the network, analyses the collected data, and then provides actionable insights to any other network function that is a consumer of that data. Since the NWDAF is a disaggregated network virtual function, this might at first ring a bell about the internally competing SON systems of 4G. However, it is not expected to be the case due to the standardized way of exposing data in a virtualized cloud environment and the ability to orchestrate functions in the 5G network to a much higher degree than in traditional networks. As such, the distributed NWDAF function can apply the AI-based logic coherently and in coordination with all parts of the network, the core, the edge, and the radio network. NWDAF is more akin to an octopus’s nervous system, which has a central brain and autonomous arms working in coordination. Some aspects of the operation would benefit from a local and low-latency operation at the network edge (the arms of the octopus autonomously grab and feel objects).
In contrast, others need a centralized approach to avoid conflicts between simultaneous optimization efforts (like the graceful movement of the whole body of the octopus in a direction). In other words, the analytics should be available where needed, whether the service is about URLLC (ultra-reliable-low-latency) communication at the edge or load-balancing resources for various service applications. The design and architecture of the 5G network can do just that.
Use Case Scenario
As an example, the NWDAF can be deployed to monitor real-time traffic analysis for predicting events as they occur in the network. One challenge is mass events at venues, which can be attended by hundreds of thousands of subscribers or more for a sudden and short span of time. Dimensioning network resources for such scenarios is costly and difficult, even in the best-case scenario when the service provider knows when the event will occur. Still, such events can occur with short notice or even spontaneously, making real-time response necessary. Now, partitioning resources in the network is enabled by Network Slicing, but exactly how and when resources need to be sliced and used will be based on the NWDAF analytics and predictive capabilities. For example, the sequence of events could follow something like this:
1) The core nodes AMF/SMF/UPF keep feeding a central NWDAF with real-time data on traffic.
2) The NWDAF carries out data analytics based on AI algorithms on the continuous data stream from these core nodes and predicts a quick rise in traffic demands in a specific area. The predictive power of the AI algorithm is trained and enhanced by models from past experiences with such events. This data can also be analyzed by a distributed network of NWDAFs.
3) The NWDAF distributes actionable insights to the nodes at the edge and the radio network to take appropriate action for managing the load and meeting a dramatic increase in demand, implying throttling down data rates, load-balancing aggressively across multiple frequency bands, or even instantiation of new virtual nodes to handle the required load.
Summary
With the advent of 5G, the expected exponential growth in the Internet of Things and the data it can generate, and the parallel rapid developments in artificial intelligence and machine learning, the wireless telecom industry has an exceptional opportunity to turn massive amounts of data into actionable, productive insights. Indeed, it is generally agreed that the impetus for the enormous gains we have seen in AI and ML, as in ChatGPT and DallE, was primarily due to the availability of Big Data, applied to the decades-old concept of neural networks. The NWDAF and its variations may enable the same quantum leap in managing and operating 5G wireless networks of today and tomorrow by harnessing the enormous untapped resources hidden in network-generated data.